Calculating Moving Averages and Historical Flow Quantiles
Using the R-packages dataRetrieval, dplyr, and ggplot2, a simple discription on how to create a moving-average plot with historical flow quantiles.
This post will show simple way to calculate moving averages, calculate historical-flow quantiles, and plot that information. The goal is to reproduce the graph at this link: PA Graph . The motivation for this post was inspired by a USGS colleague that that is considering creating these type of plots in R. We thought this plot provided an especially fun challenge - maybe you will, too!
First we get the data using the dataRetrieval package. The siteNumber and parameterCd could be adjusted for other sites or measured parameters. In this example, we are getting discharge (parameter code 00060) at a site in PA.
It may be important to note that this script is a bit lazy in handling leap days.
Get data using dataRetrieval
Calculate moving average
Next, we calculate a 30-day moving average on all of the flow data:
Calculate historical percentiles
We can use the quantile
function to calculate historical percentile flows. Then use the loess
function for smoothing. The argument smooth.span
defines how much smoothing should be applied. To get a smooth transistion at the start of the graph, we can add include an earlier year which is not plotted at the end.
Plot using base R
Many of the graphical requirements defined by the USGS are difficult to achieve in ggplot2
. Base R plotting can be used to obtain these types of graphs:
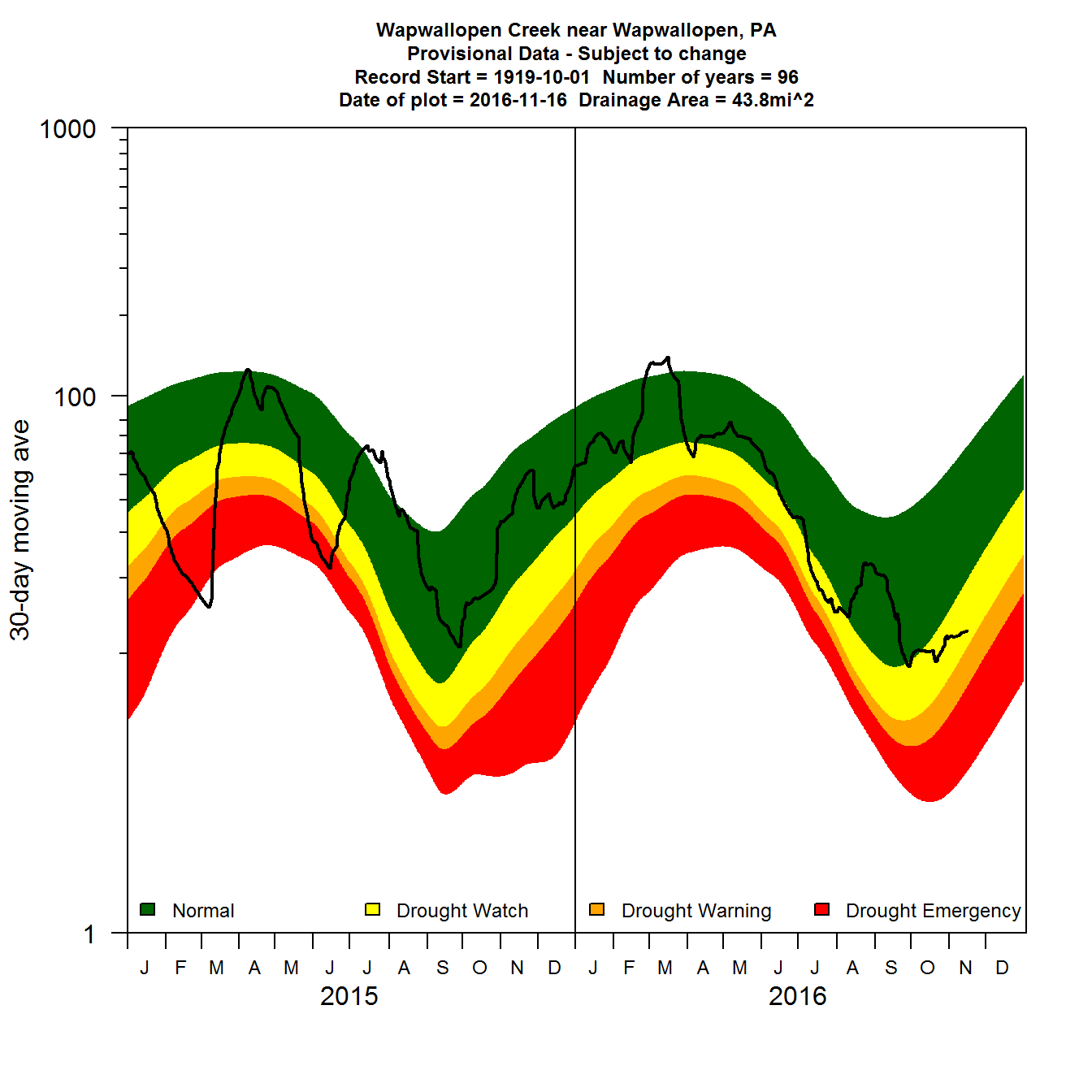
Simple 30-day moving average daily flow plot using base R
Plot using ggplot2
Finally, we can also try to create the graph using the ggplot2
package. The following script shows a simple way to re-create the graph in ggplot2
with no effort on imitating desired style:
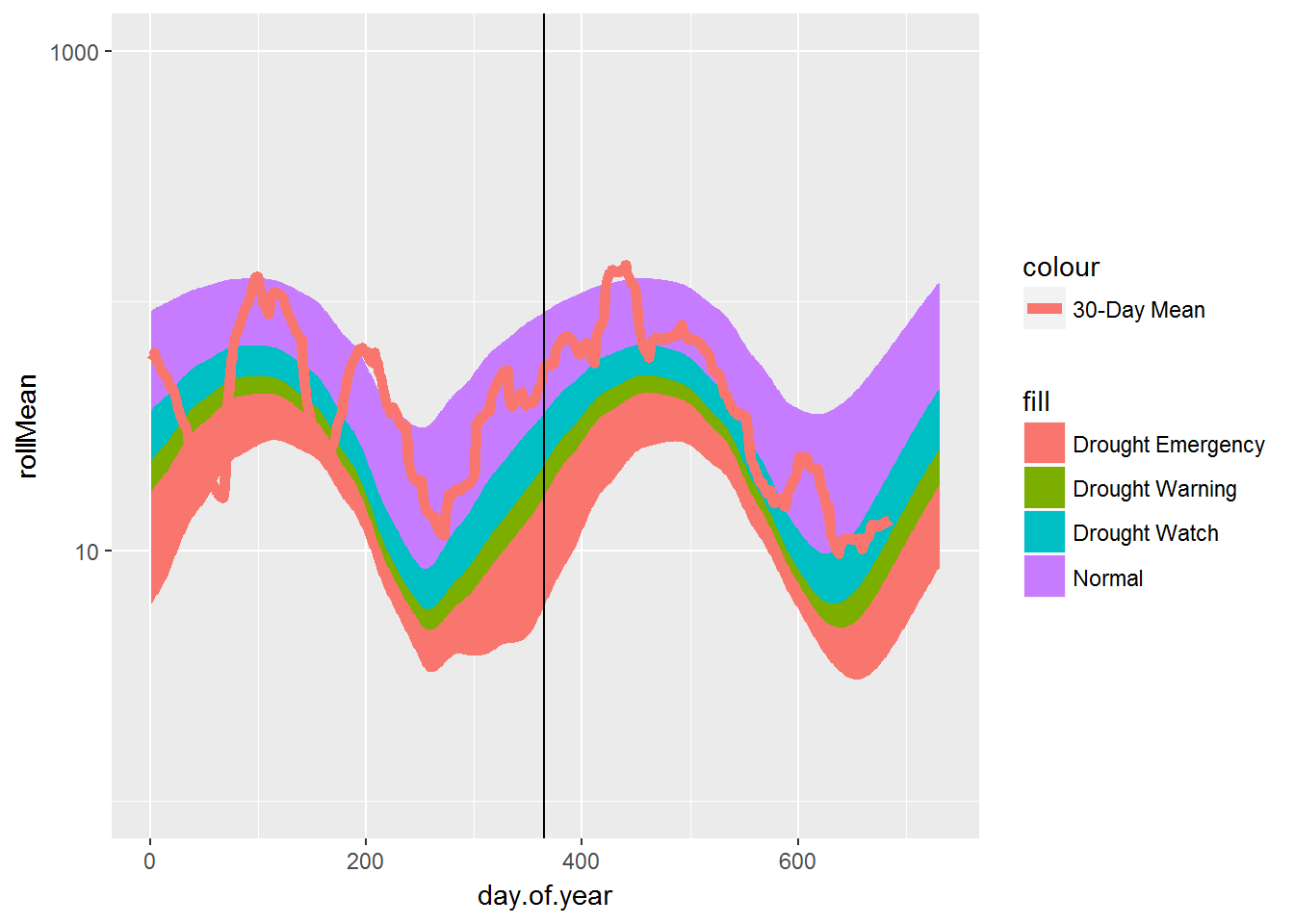
Simple 30-day moving average daily flow plot using ggplot2
Next, we can play with various options to do a better job to imitate the style:
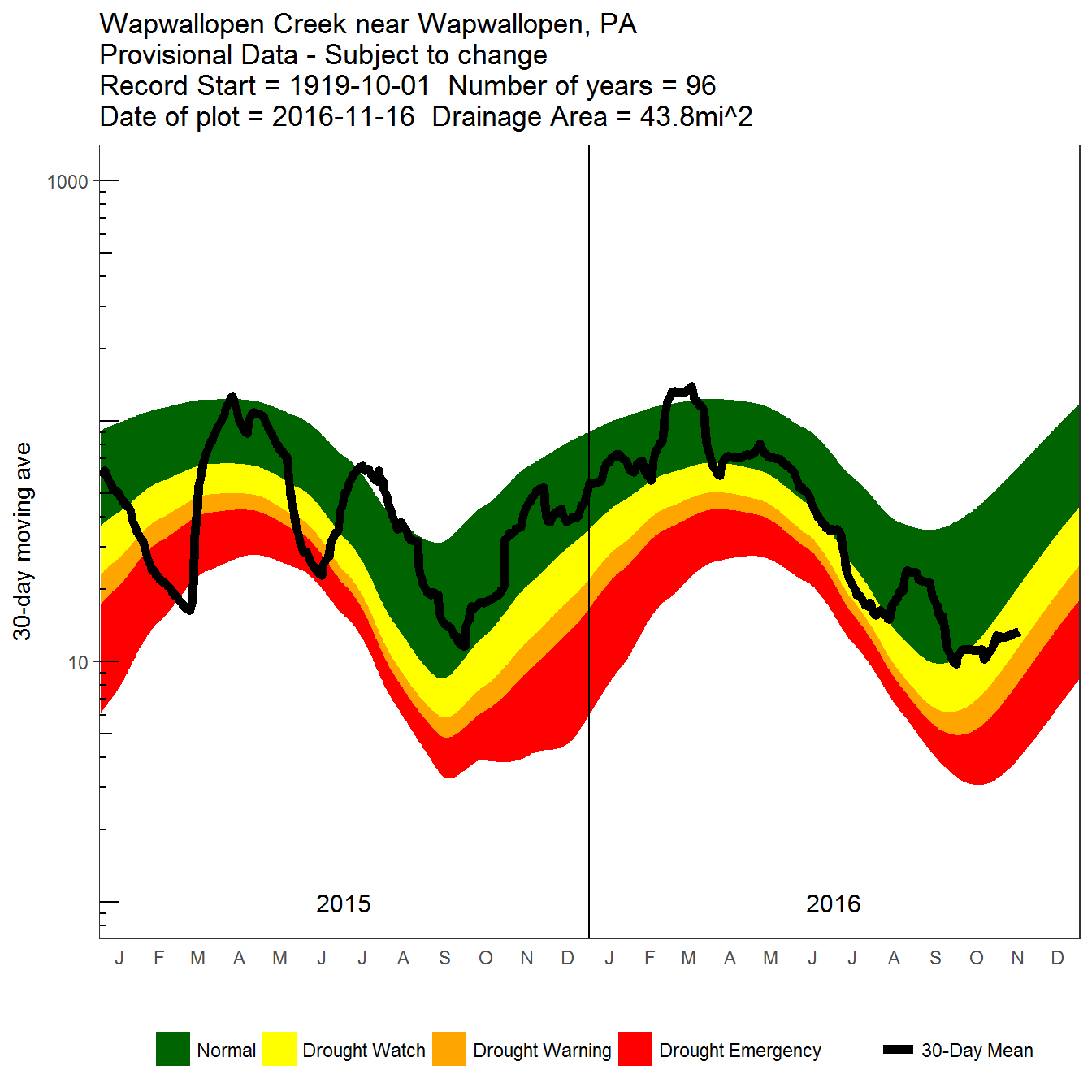
Detailed 30-day moving average daily flow plot
Questions
Please direct any questions or comments on dataRetrieval
to: https://github.com/DOI-USGS/dataRetrieval/issues
Categories:
Keywords:
Related Posts
Using the dataRetrieval Stats Service
October 5, 2016
Introduction This script utilizes the new dataRetrieval package access to the USGS Statistics Web Service . We will be pulling daily mean data using the daily value service in readNWISdata, and using the stats service data to put it in the context of the site’s history.
Using Leaflet and htmlwidgets in a Hugo-generated page
August 23, 2016
We are excited to use many of the JavaScript data visualizations in R using the htmlwidgets package in future posts. Having decided on using Hugo , one of our first tasks was to figure out a fairly straightforward way to incorporate these widgets.
Reproducible Data Science in R: Iterate, don't duplicate
July 18, 2024
Overview This blog post is part of a series that works up from functional programming foundations through the use of the targets R package to create efficient, reproducible data workflows.
Reproducible Data Science in R: Writing better functions
June 17, 2024
Overview This blog post is part of a series that works up from functional programming foundations through the use of the targets R package to create efficient, reproducible data workflows.
Reproducible Data Science in R: Writing functions that work for you
May 14, 2024
Overview This blog post is part of a series that works up from functional programming foundations through the use of the targets R package to create efficient, reproducible data workflows.